Introduction to Exponential Kernel Convolution
In the consistently developing field of AI and sign handling, dramatic piece exponential kernel convolution stands apart as a urgent idea. It is instrumental in smoothing information and improving the exactness of different computational models. This article dives into the complexities of remarkable bit convolution, revealing insight into its applications, numerical establishments, and pragmatic ramifications.
What is Exponential Kernel Convolution?
Exponential kernel convolution is a method used to smooth or filter signals and data points in a way that emphasizes recent values more heavily than older ones.This method use the properties of the remarkable part, which is characterized numerically as:
K(x)=e−λ∣x∣K(x) = e^{-\lambda |x|}K(x)=e−λ∣x∣
where λ\lambdaλ is a positive boundary that controls the rot pace of the bit. The portion capability K(x)K(x)K(x) decides how much impact a specific information point has in view of its separation from the middle.
Mathematical Foundations of Exponential Kernel Convolution
The course of exponential kernel convolution with a dramatic bit includes coordinating the result of the portion capability and the sign over every conceivable shift. Officially, on the off chance that f(x)f(x)f(x) is the capability to be convolved and K(x)K(x)K(x) is the outstanding piece, the convolution g(x)g(x)g(x) is given by:
g(x)=(f∗K)(x)=∫−∞∞f(t)K(x−t) dtg(x) = (f * K)(x) = \int_{-\infty}^{\infty} f(t) K(x – t) \, dtg(x)=(f∗K)(x)=∫−∞∞f(t)K(x−t)dt
In the context of the exponential kernel, this equation becomes:
g(x)=∫−∞∞f(t)e−λ∣x−t∣ dtg(x) = \int_{-\infty}^{\infty} f(t) e^{-\lambda |x – t|} \, dtg(x)=∫−∞∞ f(t)e−λ∣x−t∣dt
This integral calculates the weighted average of the function f(t)f(t)f(t), with weights decreasing exponentially as the distance between xxx and ttt increases.
Applications of Exponential Kernel Convolution
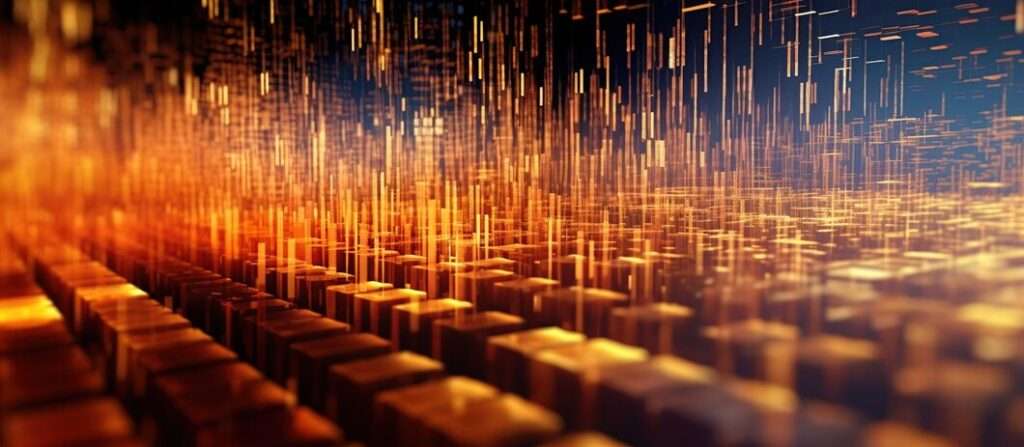
1. Signal Smoothing
One of the primary uses of exponential kernel convolution is in signal smoothing. In digital signal processing, this technique helps in reducing noise and making the underlying signal more discernible. The exponential kernel‘s ability to assign higher weights to more recent data points ensures that the smoothed signal accurately reflects the latest trends.
2. Time Series Analysis
In time series assessment, emotional part convolution is used to apply exceptional moving midpoints. This approach is extensively used in financial business areas to follow examples and make conjectures. By applying a striking piece, inspectors can filter through transient changes and focus on long stretch examples.
3. Image Processing
In the domain of picture handling, outstanding portion exponential kernel convolution is utilized for obscuring and edge discovery. The dramatic piece can streamline the commotion in a picture while protecting the significant advantages and designs. This makes it a significant device in PC vision applications where it is vital to keep up with edge clearness.
4. Machine Learning Models
In AI, remarkable part convolution upgrades the presentation of calculations by preprocessing information. It is particularly valuable in relapse and characterization undertakings where smoothing information can prompt better model speculation and decreased overfitting.
Advantages of Exponential Kernel Convolution
1. Adaptive Smoothing
The remarkable portion’s capacity to adaptively smooth information in light of late qualities makes it especially viable in powerful conditions where patterns change quickly. Not at all like different parts, which might apply uniform smoothing across all significant pieces of information, the remarkable bit guarantees that the most important information focuses are given more weight.
2. Efficient Computation
Figuring the convolution utilizing a remarkable piece is somewhat productive because of the part’s straightforward numerical structure. This effectiveness is profitable continuously applications where speedy handling is fundamental.
3. Flexibility
The rot boundary λ\lambdaλ in the outstanding portion considers adaptability in changing the smoothing impact. By tuning λ\lambdaλ, professionals have some control over the degree of smoothing and tailor the cycle to explicit necessities and applications.
Challenges and Considerations
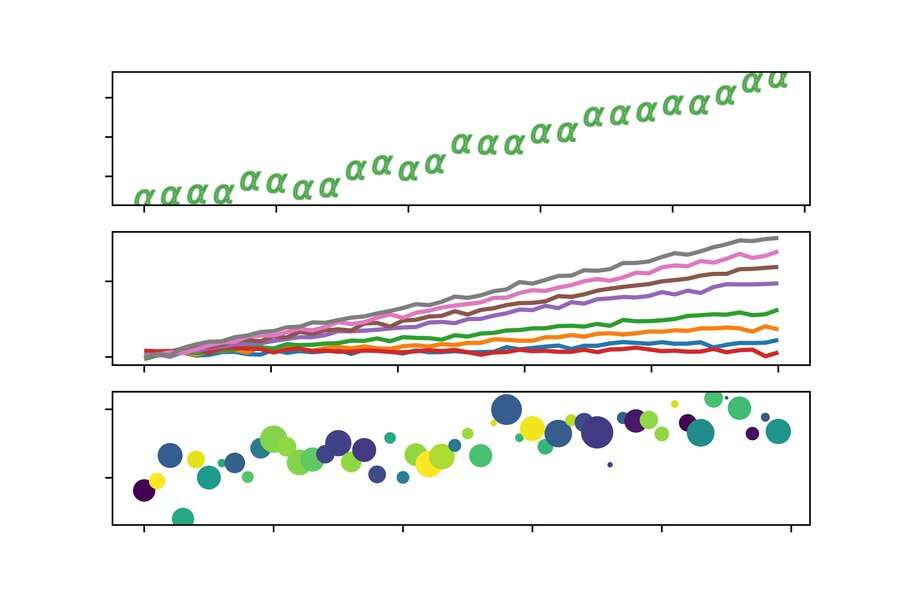
1. Parameter Selection
Picking the proper rot boundary λ\lambdaλ is pivotal. Assuming λ\lambdaλ is too enormous, the portion may excessively smooth the information, bringing about loss of significant data. Alternately, assuming λ\lambdaλ is too little, the smoothing impact might be lacking, leaving clamor in the information.
2. Boundary Effects
In convolution activities, limit impacts can now and again mutilate results, particularly close to the edges of the information. Cautious treatment of limits is important to relieve these impacts and guarantee precise outcomes.
3. Computational Complexity
While the outstanding portion improves on calculations contrasted with additional intricate parts, exponential kernel convolution tasks can in any case be computationally escalated for huge datasets. Improving calculations and utilizing productive processing assets can assist with tending to this test.
Implementation Techniques
1. Discrete Convolution
For reasonable applications, discrete exponential kernel convolution is much of the time utilized, particularly in advanced signal handling. This includes approximating the persistent convolution basic with a summation over discrete pieces of information.
2. Fast Algorithms
To further develop productivity, quick calculations, for example, the Quick Fourier Change (FFT) can be utilized. These calculations decrease the computational intricacy of exponential kernel convolution activities, making them appropriate for huge scope applications.
Conclusion
Outstanding portion exponential kernel convolution is an amazing asset with a large number of utilizations across signal handling, time series examination, picture handling, and AI. Its capacity to adaptively smooth information and its effective computational properties make it an important method in different fields. By figuring out its numerical establishments, benefits, and pragmatic contemplations, experts can successfully use dramatic piece convolution to improve their information examination and handling assignments.